The modern tourism industry in the 21st century faces numerous challenges. One of these challenges is the rapidly growing number of tourists in spatially constrained areas such as historic city centers, museums, or geographical bottlenecks like narrow valleys. In this context, accurate and reliable prediction of tourism volume and flow within a specific area is crucial for visitor management tasks such as controlling visitor flow and avoiding overcrowding. Static flow control methods like limiting access to hotspots or using conventional low-level controllers have thus far been unable to solve the problem. In this study, we empirically evaluate the performance of several advanced deep learning methods in the field of limited data visitor flow prediction. We utilize granular data provided by a tourism region and compare the results with ARIMA, a classical statistical method. Our findings demonstrate that deep learning models outperform the ARIMA method in terms of prediction accuracy, while both exhibit faster inference times and the ability to incorporate additional input features.
For further information on our published paper, please refer to https://arxiv.org/abs/2206.13274
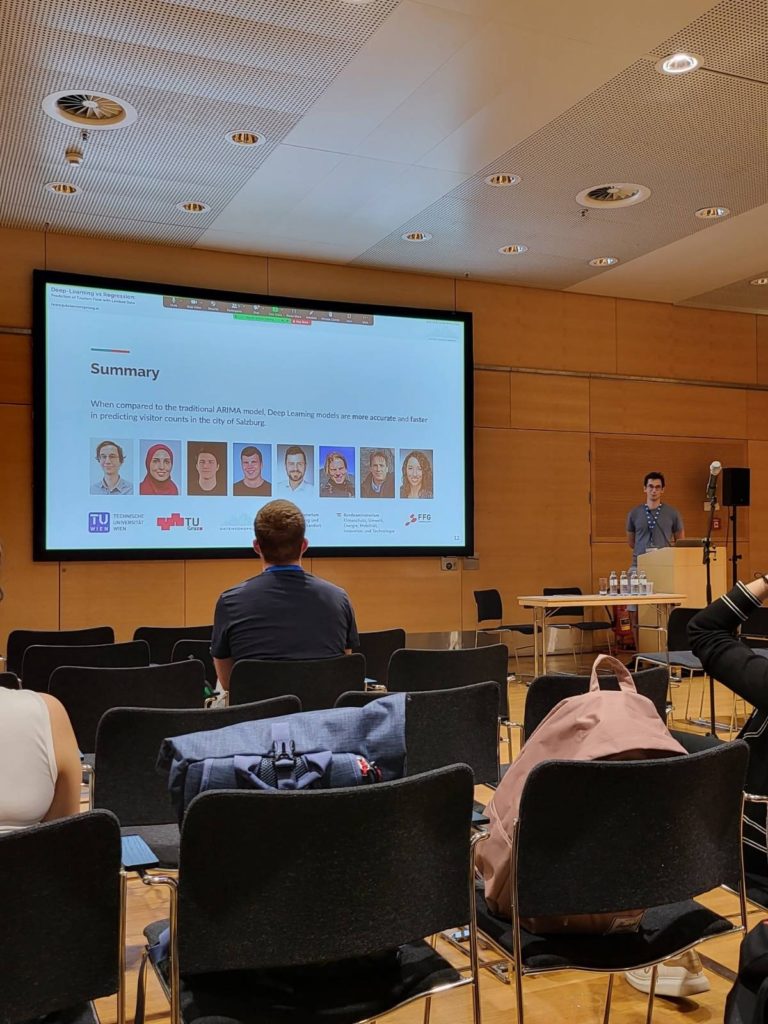